Ivanka Vasenska – South-West University “Neofit Rilski”, 60 Ivan Mihaylov str., Bulgaria
Keywords:
Time series;
Deep machine learning;
Artificial intelligence;
Bulgaria inbound tourism
forecast
DOI: https://doi.org/10.31410/ITEMA.2023.73
Abstract: Accurate inbound tourism flow forecasting has been a challenge for all stakeholders related to the sector. The multidisciplinary character of the tourism product which has been directly and indirectly influenced by all types of risks, cataclysms and crises further exposed its intangible nature to shocks and flows disruption. Thus, forecasting inbound tourism flows with advanced data science and AI (artificial intelligence) methods has been gaining momentum, which the COVID-19 pandemic boosted. Therefore, this paper aims to examine the relevant AI forecasting methods by applying a deep machine learning technique comparing different Python time series forecasting libraries via a Jupyter Notebook computer environment. Bulgaria’s inbound tourism data has been used to develop an advanced deep neural network with the DARTS Python library and compare its accuracy with other Python library models.
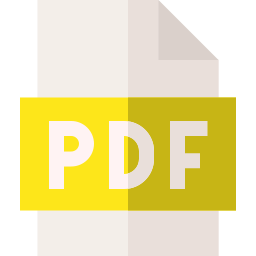
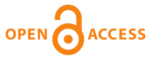
7th International Scientific Conference on Recent Advances in Information Technology, Tourism, Economics, Management and Agriculture – ITEMA 2023 – Conference Proceedings, Hybrid (Faculty of Organization and Informatics Varaždin, University of Zagreb, Croatia), October 26, 2023
ITEMA Conference Proceedings published by: Association of Economists and Managers of the Balkans – Belgrade, Serbia
ITEMA conference partners: Faculty of Economics and Business, University of Maribor, Slovenia; Faculty of Organization and Informatics, University of Zagreb, Varaždin; Faculty of Geography, University of Belgrade, Serbia; Institute of Marketing, Poznan University of Economics and Business, Poland; Faculty of Agriculture, Banat’s University of Agricultural Sciences and Veterinary Medicine ”King Michael I of Romania”, Romania
ITEMA Conference 2023 Conference Proceedings: ISBN 978-86-80194-75-2, ISSN 2683-5991, DOI: https://doi.org/10.31410/ITEMA.2023
Creative Commons Non Commercial CC BY-NC: This article is distributed under the terms of the Creative Commons Attribution-Non-Commercial 4.0 License (https://creativecommons.org/licenses/by-nc/4.0/) which permits non-commercial use, reproduction and distribution of the work without further permission.
References
Copeland, B. (2023, October 11). Artificial intelligence. Encyclopedia Britannica. https://www.britannica.com/technology/artificial-intelligence
Egger, R. (2022). Applied Data Science in Tourism. (R. Egger, Edd.) Springer Nature. https://doi.org/10.1007/978-3-030-88389-8
Eurostat. (2023). Arrivals at tourist accommodation establishments – monthly data. Retrieved from Tourism Industries – monthly data: https://ec.europa.eu/eurostat/databrowser/view/TOUR_OCC_ARM__custom_6400112/default/table
Géron, A. (2019). Hands-On Machine Learning with Scikit-Learn and TensorFlow: Concepts, Tools, and Techniques to Build Intelligent Systems (Vol. 1). O’Reilly Media. ISBN: 9781492032649
Goodfellow, I., Bengio, Y., & Courville, A. (2016). Deep Learning. MIT Press. ISBN-13: 978-0262035613
Gretzel, U., Fuchs, M., Baggio, R., Hoepken, W., Law, R., Neidhardt, J., Pesonen, J., Zanker, M., & Xiang, Z. (2020). e-Tourism beyond COVID-19: a call for transformative research. Information Technology & Tourism, 22(2), 187-203. https://doi.org/10.1007/s40558-020-00181-3
Herzen, J., Lässig, F., Piazzetta, S. G., Neuer, T., Tafti, L., Raille, G., & Grosh, .. &. (2022). Darts: User-Friendly Modern Machine Learning for Time Series. The Journal of Machine Learning Research, 23(1), 5442-5447. Retrieved from https://unit8co.github.io/darts/
Lazzeri, F. (2021). Python Open Source Libraries for Scaling Time Series Forecasting Solutions. Data Science at Microsoft. https://medium.com/data-science-at-microsoft/python-open-source-libraries-for-scaling-time-series-forecasting-solutions-3485c3bd8156
McCulloch, W. S., & Pitts, W. (1943). A logical calculus of the ideas immanent in nervous activity. The bulletin of mathematical biophysics, 5. https://doi.org/10.1007/BF02478259
Mich, L. (2020). Artificial Intelligence and Machine Learning. Handbook of e-Tourism, 1-21. https://doi.org/10.1007/978-3-030-05324-6_25-1
The National Statistical Institute of the Republic of Bulgaria. (2023). Tourism. Retrieved from Business statistics: https://www.nsi.bg/en/content/1847/tourism
Neuburger, L., Beck, J., & Egger, R. (2018). Chapter 9 The ‘Phygital’ Tourist Experience: The Use of Augmented and Virtual Reality in Destination Marketing. Tourism Planning and Destination Marketing, 183-202. https://doi.org/10.1108/978-1-78756-291-220181009
Provost, F., & Fawcett, T. (2013). Data Science and its Relationship to Big Data and Data-Driven Decision Making. Big Data, 1(1), 51-59. https://doi.org/10.1089/big.2013.1508
Skiena, S. S. (2017). The Data Science Design Manual. Texts in Computer Science. https://doi.org/10.1007/978-3-319-55444-0
Turing, A. M. (1936). On computable numbers, with an application to the Entscheidungsproblem. Journal of Math, 58 (345-363), 5.